Bynder’s Face Recognition capabilities
Bynder’s Face Recognition is a powerful solution designed for organizations working with large volumes of visual content. This feature allows users to automatically identify faces within images and tag them in bulk—simplifying the process of organizing and retrieving images of specific people whenever needed.
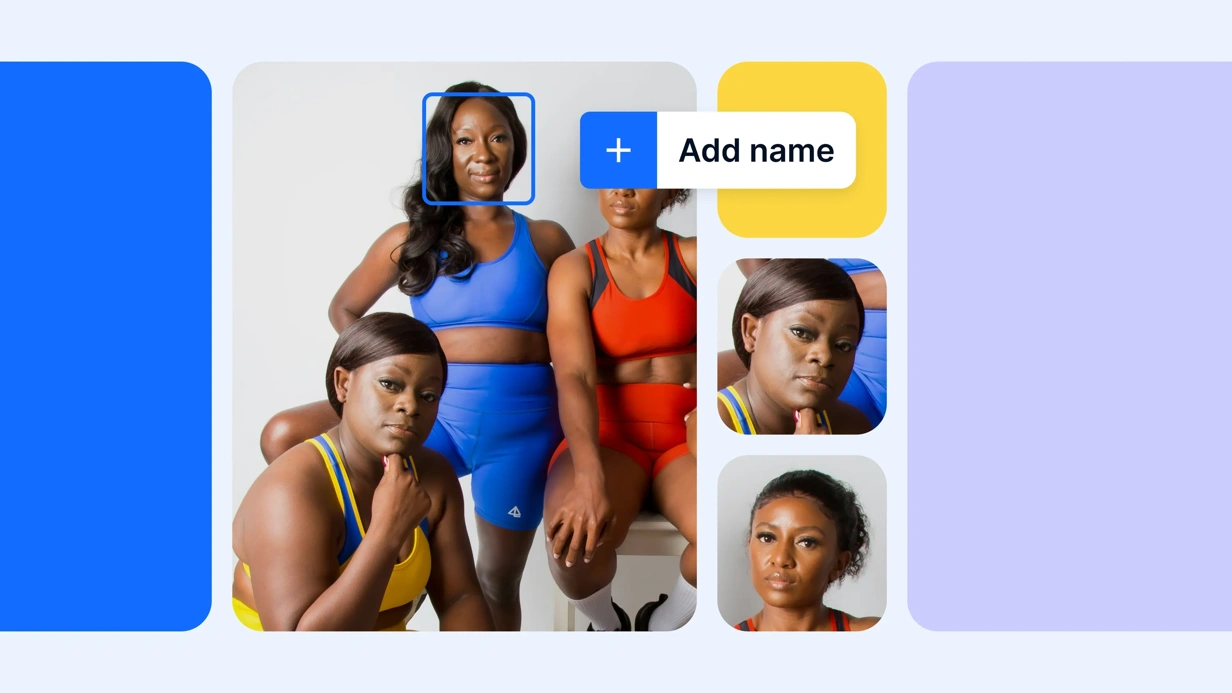
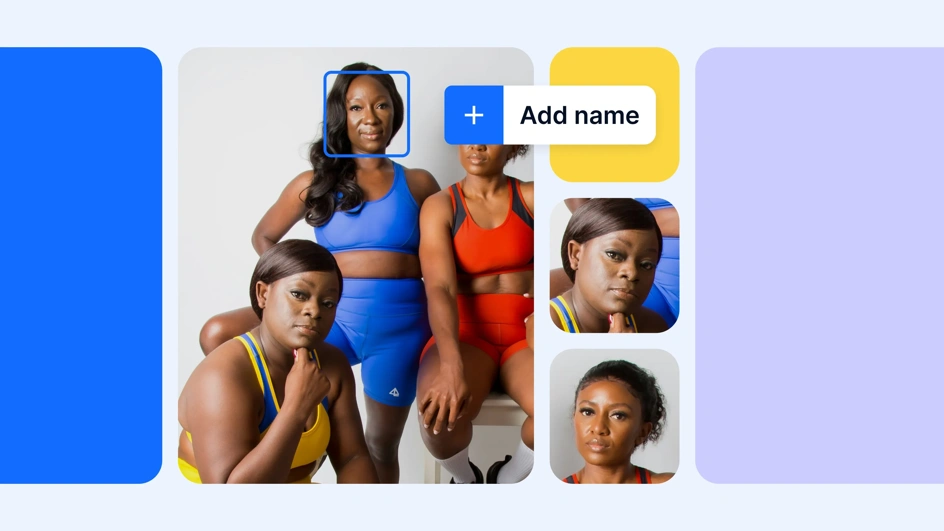
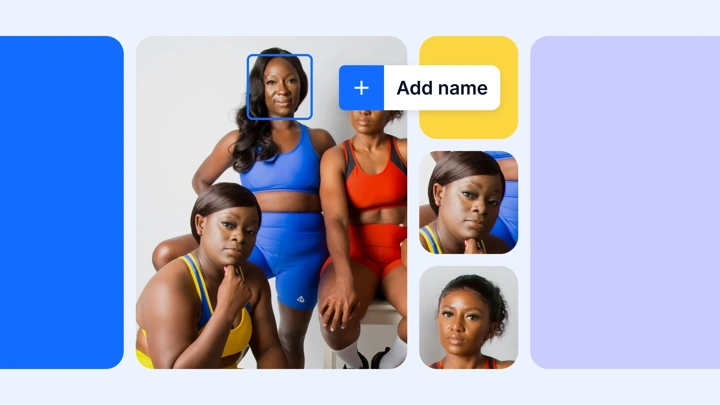
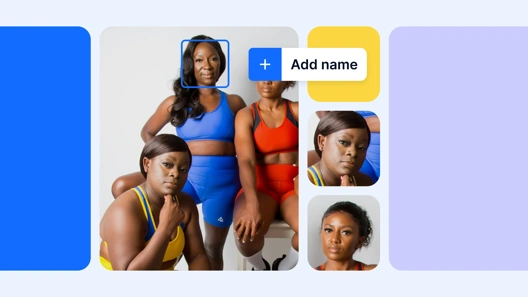
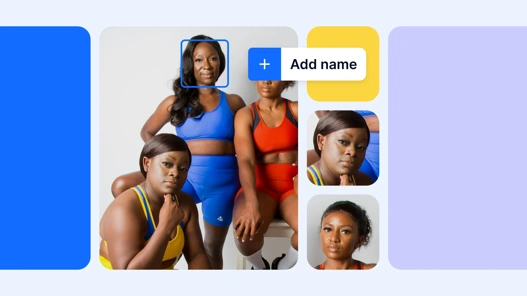
Understanding Face Recognition in digital asset management systems
DAM systems process vast quantities of visual content daily, with a significant portion containing people. As organizations scale their visual content operations, the need for automated image analysis becomes crucial. Face recognition technology, powered by deep learning algorithms, has emerged as a transformative solution to this challenge, enabling organizations to streamline workflows and maintain better control over their digital assets.
A brief history of face recognition technology
Face recognition technology originated in the 1960s when early computer scientists developed rudimentary systems to identify faces based on manual feature mapping. By the 1990s, algorithms like Eigenfaces introduced statistical analysis methods that made face recognition more reliable. The advent of deep learning in the 2010s revolutionized the field, allowing models to analyze complex patterns and achieve unprecedented accuracy.
Cloud-based platforms like Amazon Rekognition highlight the advancements in face recognition technology. With scalable infrastructure and neural networks, Rekognition offers accurate recognition capabilities, trained on millions of images to detect and analyze faces reliably even in difficult conditions like poor lighting or partial visibility.
Technical foundation
Face recognition in DAM systems operates through sophisticated cloud-based image analysis services that leverage deep learning technology. These services process images through multiple stages:
- Face detection: The system scans images to locate all detectable faces.
- Facial analysis: Distinct facial features are analyzed to generate unique feature vectors.
- Face matching and recognition: These feature vectors are compared against stored patterns to identify or suggest potential matches
This process eliminates the need for end users to have machine learning expertise while delivering high accuracy and scalability. Once faces are detected and analyzed, the results are seamlessly integrated into the DAM’s metadata system, enabling instant searchability across the asset library.
Implementation in DAM systems
When implemented in a DAM environment, face recognition creates an automated workflow that continuously processes new uploads. The system identifies faces and suggests potential matches based on existing tagged individuals, while users can verify these suggestions and link faces to specific names. This creates a learning pattern that improves accuracy over time.
The technology integrates seamlessly with existing DAM workflows through smart filters and bulk processing capabilities. It enhances metadata schemas with face recognition data, maintaining consistent naming conventions across large asset libraries. This automation significantly reduces the manual effort required for organizing and retrieving images of specific individuals.
Practical applications and common use cases
Organizations across various sectors utilize face recognition in DAM for different purposes:
- Media organizations use it to quickly retrieve images of specific public figures and organize photo archives.
- Corporate communications teams leverage the technology to manage employee headshots and event photography.
- Educational institutions apply it to organize student and faculty imagery while maintaining historical photo archives.
This powerful combination of deep learning technology and practical application demonstrates how artificial intelligence continues to transform how organizations manage and utilize their digital assets, making content more accessible and easier to manage at scale.
Future developments in this field will likely focus on enhanced accuracy through advanced machine learning algorithms, more efficient processing methods, and improved integration with other AI-powered features. As the technology matures, we can expect to see even more sophisticated applications that further streamline digital asset management workflows.
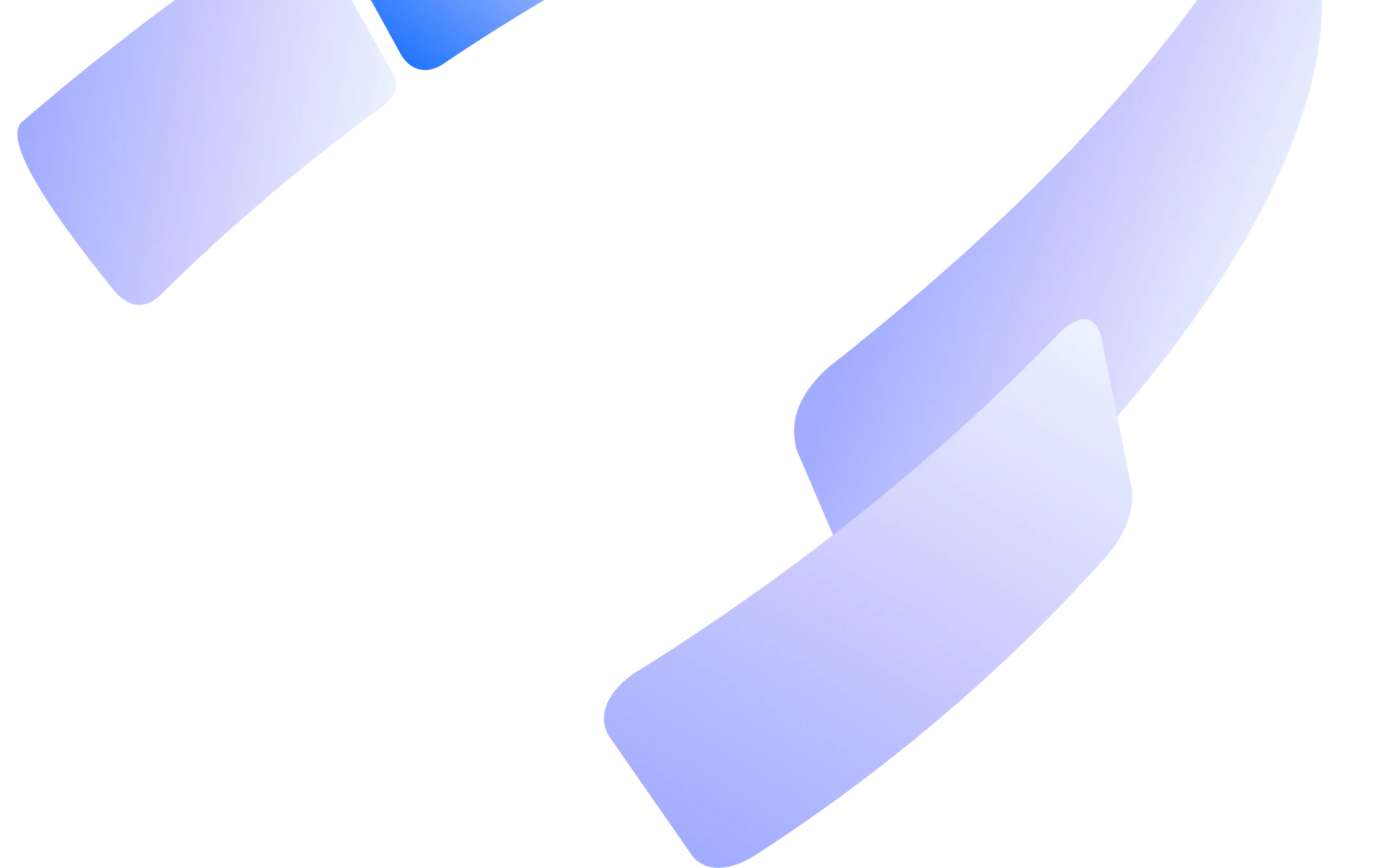